
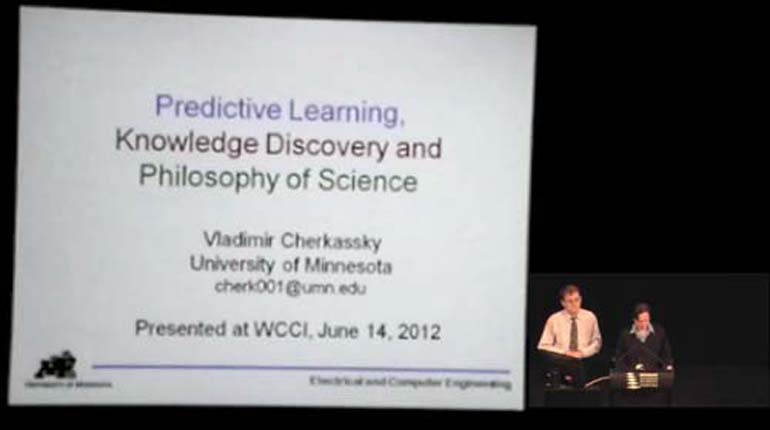
Vladimir Cherkassky - Predictive Learning, Knowledge Discovery and Philosophy of Science
Abstract: Various disciplines, such as machine learning, statistics, data mining and artificial neural networks, are concerned with the estimation of data-analytic models. A closer inspection reveals that a common theme among all these methodologies is estimation of predictive models from data. In our digital age, an abundance of data and cheap computing power offers hope of knowledge discovery via application of statistical and machine learning algorithms to empirical data. This data-analytic knowledge has both similarities and differences with classical first-principle scientific knowledge. For example, any scientific theory can be viewed as inductive theory because it generalizes over a finite number of observations (or experiments). Thus, the problems of induction and knowledge discovery have been thoroughly investigated in Western philosophy of science. This philosophical analysis dates back to Kant and Hume who investigated the problem of logical induction, as well as psychological induction. Any knowledge involves a combination of hypotheses/ideas and empirical data. In the modern digital age, the balance between ideas (mental constructs) and observed data (facts) has completely shifted. Classical scientific knowledge was produced mainly by a stroke of genius (e.g., Newton, Maxwell, Einstein). In contrast, much of modern knowledge in life sciences and social sciences is derived via data-analytic modeling. This data-driven knowledge is obtained following the VC-theoretical methodological framework, also known as predictive learning. This paper presents a brief survey of the philosophical concepts related to inductive inference, and then extends these ideas to predictive data-analytic knowledge discovery. Further, we relate these classical philosophical ideas to modern statistical learning. Finally, we apply the philosophical and methodological framework of predictive learning to interpretation of data-analytic models, using application examples from financial engineering and life sciences.
Abstract: Various disciplines, such as machine learning, statistics, data mining and artificial neural networks, are concerned with the estimation of data-analytic models. A closer inspection reveals that a common theme among all these methodologies is estimation of predictive models from data. In our digital age, an abundance of data and cheap computing power offers hope of knowledge discovery via application of statistical and machine learning algorithms to empirical data. This data-analytic knowledge has both similarities and differences with classical first-principle scientific knowledge. For example, any scientific theory can be viewed as inductive theory because it generalizes over a finite number of observations (or experiments). Thus, the problems of induction and knowledge discovery have been thoroughly investigated in Western philosophy of science. This philosophical analysis dates back to Kant and Hume who investigated the problem of logical induction, as well as psychological induction. Any knowledge involves a combination of hypotheses/ideas and empirical data. In the modern digital age, the balance between ideas (mental constructs) and observed data (facts) has completely shifted. Classical scientific knowledge was produced mainly by a stroke of genius (e.g., Newton, Maxwell, Einstein). In contrast, much of modern knowledge in life sciences and social sciences is derived via data-analytic modeling. This data-driven knowledge is obtained following the VC-theoretical methodological framework, also known as predictive learning. This paper presents a brief survey of the philosophical concepts related to inductive inference, and then extends these ideas to predictive data-analytic knowledge discovery. Further, we relate these classical philosophical ideas to modern statistical learning. Finally, we apply the philosophical and methodological framework of predictive learning to interpretation of data-analytic models, using application examples from financial engineering and life sciences.